IEEE IJCNN/WCCI-2020 special session, Glasgow, Scotland, UK, 19-24 July 2020
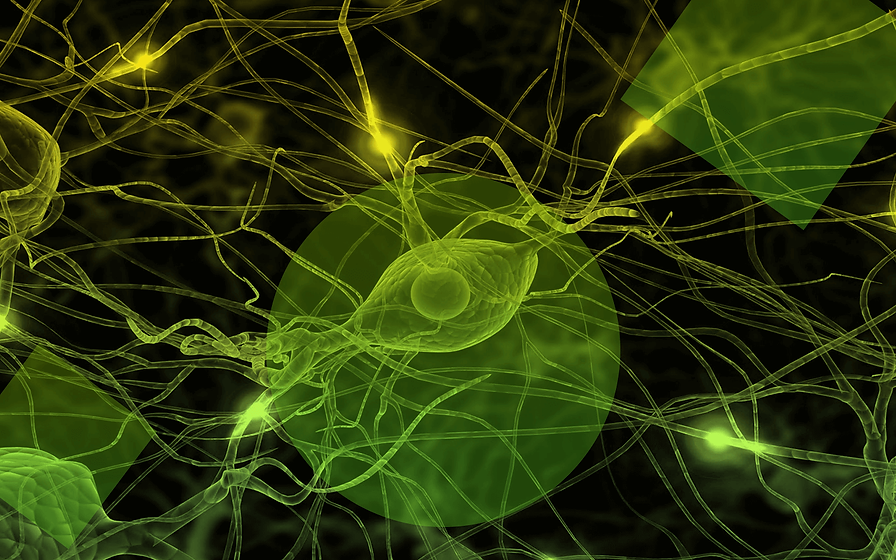
WELCOME TO IJCNN/WCCI 2020 SPECIAL SESSION ON BAYESIAN NEURAL NETWORKS
“Intelligence relies on understanding and acting in an imperfectly sensed and uncertain world” --- Prof. Zoubin Ghahramani
ABOUT BAYESIAN NEURAL NETWORKS
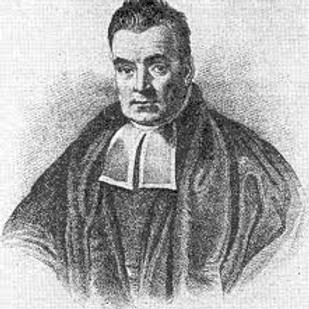
As Prof. Zoubin Ghahramani said in his Nature paper [1], “intelligence relies on understanding and acting in an imperfectly sensed and uncertain world”. Such uncertainty handling ability is even more critical for some safety-critical tasks (e.g., autonomous driving and medical diagnosis). Unfortunately, existing neural networks are weak on that. The topic of this session - Bayesian neural networks - is to combine the beauties of two fields: neural networks which is powerful on complex function approximation and hidden representation learning, and Bayesian methods which have solid theoretical foundation on uncertainty modelling. It is a newly emerging topic for neural networks. Compared to vanilla neural networks, Bayesian neural networks have distinctive advantages: 1) representing, manipulating, and mitigating uncertainty based on the solid theoretical foundations of probability; 2) encoding the prior knowledge about a problem; and 3) good interpretability thanks to its clear and meaningful probabilistic structure.
This area dates back to 1990s when Radford Neal [2], David MacKay [3], and Dayan et al. [4] used Bayesian techniques in artificial neural networks. However, there were no many following works. As recent rapid development of both neural networks and Bayesian learning over the past few years, study on Bayesian neural networks has received increasing attention, and emerged many seminal works that lay the theoretical foundation and achieve state-of-the-art performances, such as Dropout as a Bayesian approximation [5], the connection between Gaussian Process with neural networks [6], Bayesian convolutional neural networks [7], etc. To promote the development of this emerging research avenue, we organize this special session to call for investigation into new theories, models, inference algorithms, and applications of Bayesian neural networks. We believe the session will be a platform to share ideas and new results on using Bayesian approaches in neural networks and using neural networks in Bayesian modelling.
CALL FOR PAPERS
Topics
All aspects of using Bayesian approaches in neural networks and using neural networks in Bayesian modeling are welcomed, including but not limited to:
Theoretical connections between stochastic processes and neural networks;
Various prior design for different types of neural networks;
Fusion of probabilistic graphical models with neural networks (such as: neural topic models, neural stochastic processes, HMM with neural network likelihoods);
(Conditional) Variational autoencoders;
Bayesian deep learning (such deep kernel learning)
Variational Bayesian neural networks
Approximate inference for Bayesian neural networks (such as variational Bayes, expectation propagation, etc.);
Stochastic gradient Markov chain Monte Carlo (MCMC);
Scalable inference in Bayesian neural networks for big data;
Bayesian neural networks for classification and regression with uncertainty estimation;
Bayesian neural networks for transfer learning;
Bayesian neural networks for reinforcement learning;
Bayesian neural networks for causal inference;
Bayesian neural networks for temporal and spatial data mining;
Bayesian neural networks for text and web mining;
New baselines for Bayesian uncertainty in network networks.
IEEE WCCI 2020 will be held in Glasgow, Scotland, UK – one of Europe’s most dynamic cultural capitals and the “world’s friendliest city” – located in Scotland, “the most beautiful country in the world” [Rough Guides 2015, 2017]. Steeped in culture, rich in history, and alive with excitement, visitors will sense these as they walk through its elegant Victorian streets, squares, parks and gardens. IJCNN is the premier international meeting for researchers and other professionals in neural networks and related areas.
SUBMISSION
LaTeX and Word Templates
To help ensure correct formatting, please use the IEEE style files for conference proceedings as a template for your submission. These include LaTeX and Word style files.
Violations of any of the above paper specifications may result in rejection of your paper.
Click here for more information
Click here for LaTeX Template Instructions
Click here to download LaTeX Bibliography Files
Manuscript Style Information
Only papers in PDF format will be accepted.
Paper Size: US letter.
Paper Length: Each paper should have 6 to MAXIMUM 8 pages, including figures, tables and references. A maximum of two extra pages per paper is allowed (i. e., up to 10 pages), at an additional charge of $100 USD per extra page.
Paper Formatting: double column, single spaced, #10 point Times Roman font.
Margins: Left, Right, and Bottom: 0.75″ (19mm). The top margin must be 0.75″ (19 mm), except for the title page where it must be 1″ (25 mm).No page numbers please. We will insert the page numbers for you.
Note: Violations of any of the above specifications may result in rejection of your paper.
(Please do not forget to select the session name when you submitting your paper!)
Submission Link: https://ieee-cis.org/conferences/ijcnn2020/upload.php
ORGANISERS
Get to Know Us
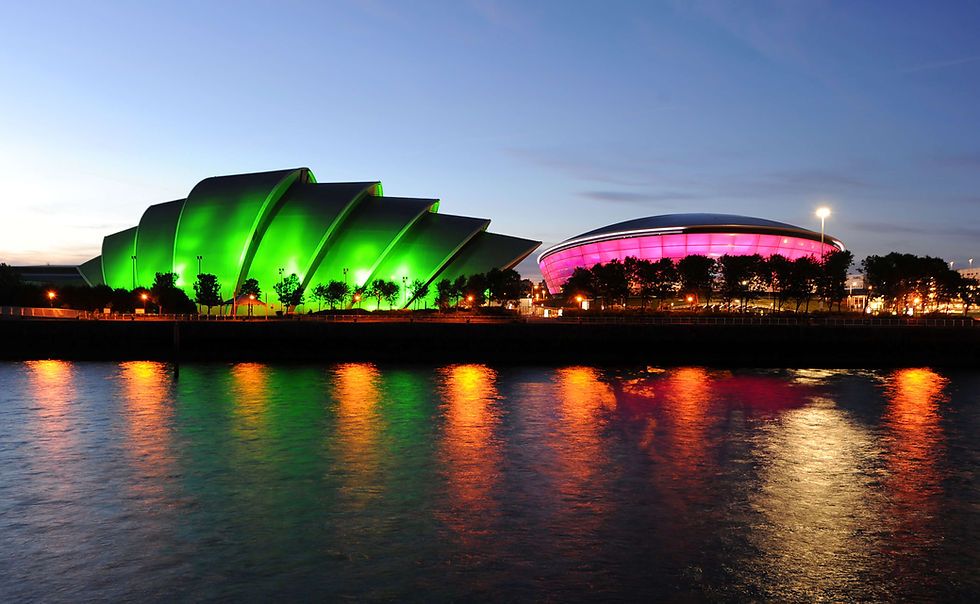
IMPORTANT DATES
15 Jan 2020 (extended to 30 Jan 2020) Paper Submission Deadline
15 Mar 2020 Paper Acceptance Notification Date
15 April 2020 Final Paper Submission and Early Registration Deadline
19-24 July 2020 IEEE WCCI 2020, Glasgow, Scotland, UK
REFERENCES
Zoubin Ghahramani, Probabilistic machine learning and artificial intelligence, Nature, 521(7553), 452, 2015.
Radford Neal, Bayesian learning for neural networks, PhD Thesis, 1995.
David MacKay, A practical Bayesian framework for backpropagation networks. Neural Computation, 4(3), 448-472, 1992.
Peter Dayan, Geoffrey Hinton, Radford Neal, and Richard Zemel. The Helmholtz machine, Neural Computation 7(5), 889-904, 1995.
Yarin Gal and Zoubin Ghahramani, Dropout as a Bayesian approximation: Representing model uncertainty in deep learning, ICML 2016.
Jaehoon Lee, et al. Deep neural networks as Gaussian processes. arXiv preprint arXiv:1711.00165, 2017.
Kumar Shridhar, Felix Laumann, and Marcus Liwicki, A Comprehensive guide to Bayesian Convolutional Neural Network with Variational Inference, arXiv preprint, arXiv: 1901.02731, 2019.